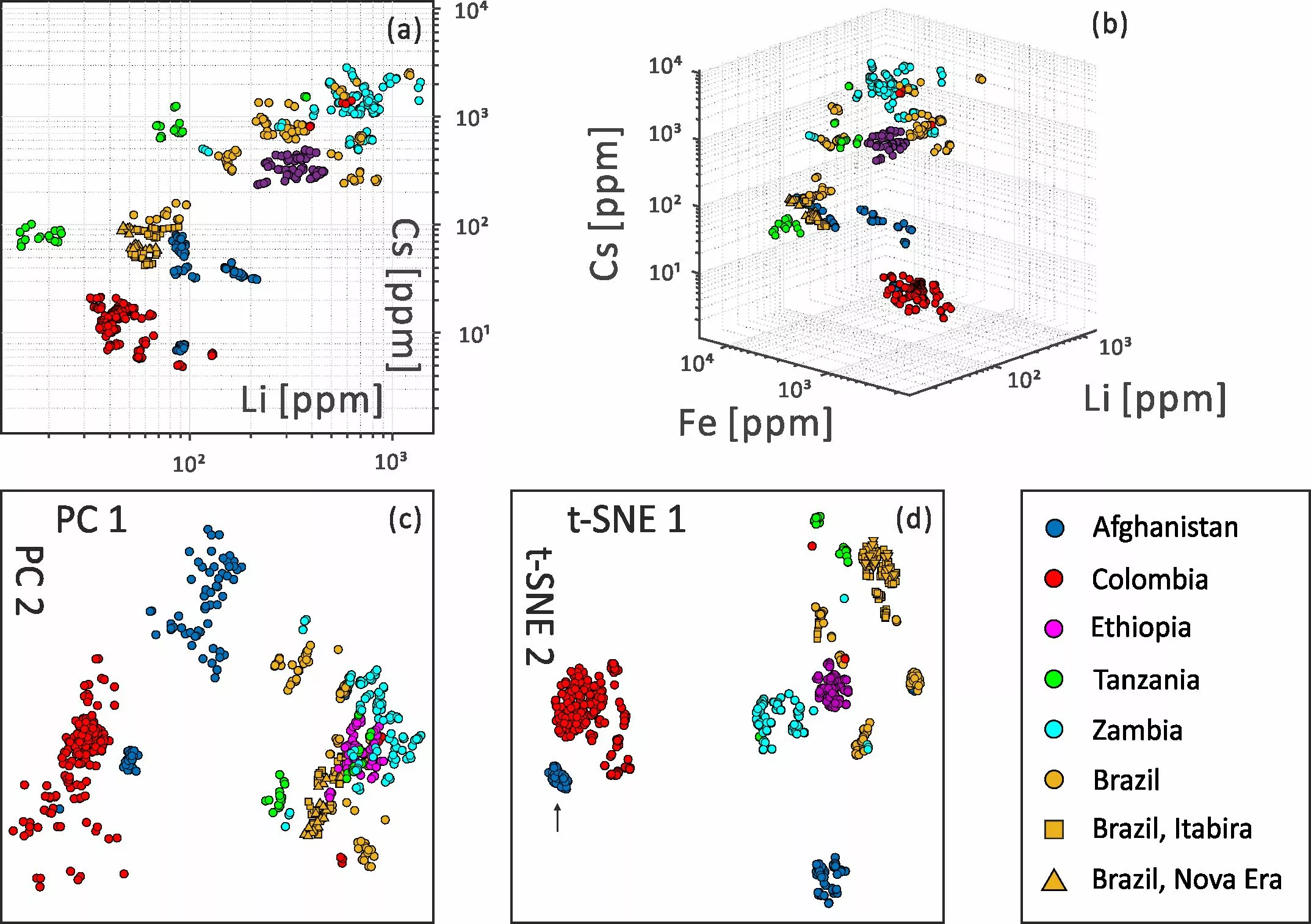
New statistical methods for analysis of gemstones
by Dr. H.A.O. Wang, first published in Facette 26 (May 2020)
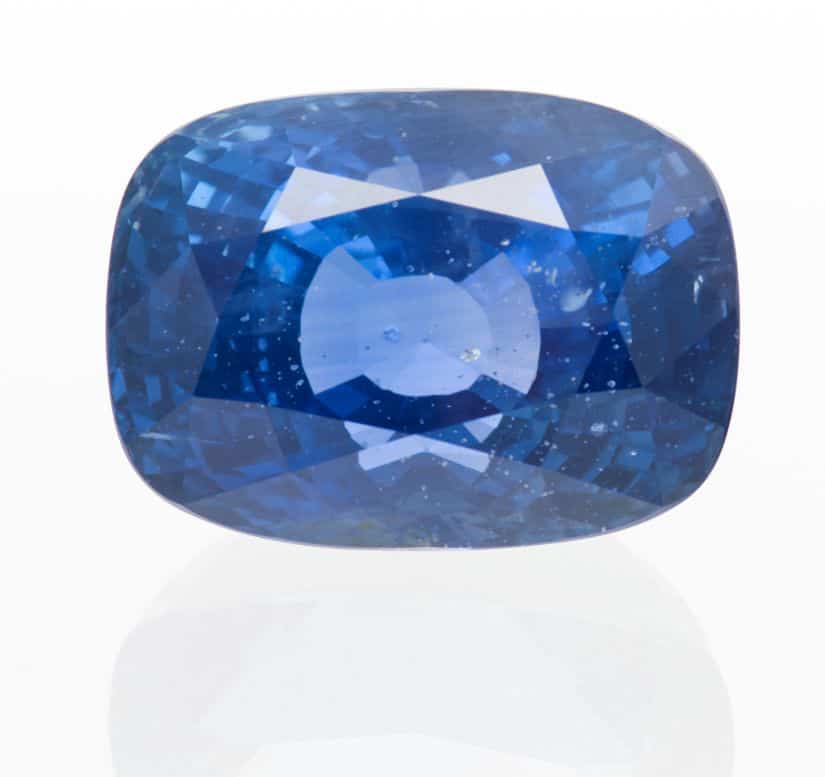
In the past decades, multi-element information has become more and more important in gem testing, not only for material identification, filtering out synthetic and treated materials, but especially for determining geological origin of gemstones. Such information is not accessible by conventional gemmological testing instruments, hence making LA-ICP-MS a unique tool in gem testing labs. At the IGC conference in Namibia in 2017, we reported a study comparing advantages and disadvantages of LA-ICP-Quadrupole-MS and LA-ICP-Time-Of-Flight- MS (LA-ICP-TOF-MS, such as GemTOF at SSEF, see in Krzemnicki et al. 2017). As described then, not only is the TOF-MS instrument capable of simultaneously acquiring almost all elements in the periodic table, it also excels in mass resolving power, which allows correction of mass interferences and improvement in quantification accuracy. In gemstone analysis, below ten parts per billion (ppb) limits of detection can be routinely achieved for heavy masses, and several hundreds of ppb for light isotopes.
LA-ICP-TOF-MS: Paradigm Shift of Multi-Element Analysis for Gemstones
During more than two years of measurements with GemTOF, the authors often encounter scenarios that a priori knowledge about multi-element content of the sample cannot be presumed, for example rarely occurring elements in gemstones, or solid or fluid inclusions in geological samples. Moreover, the isotope of interest for a specific element may also be changed in the post-data processing in case we encounter unforeseen mass interferences, which may be realized only after the measurement is done or the stone has left the premises. In this short note, we would like to revisit the advantages of TOF-MS, especially the novel acquisition scheme of FIRST measure, THEN determine which isotopes are of interest. We consider this paradigm-shift to be very useful for trace element analysis on gemstones.
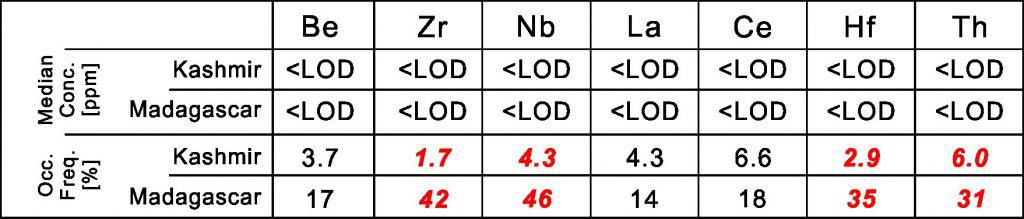
Based on real case studies on sapphire and emerald specimens, we here present how a simultaneous multi-element approach assists origin determination. Instead of pre-defining a list of isotopes in advance, routine analysis of blue sapphires using LA-ICP-TOF-MS detects rarely occurring trace elements such as beryllium (Be), zirconium (Zr), niobium (Nb), lanthanum (La), cerium (Ce), hafnium (Hf), thorium (Th). These elements have been observed more frequently in sapphires from Madagascar than Kashmir ones (Figure 1). Interestingly, radioactive thorium isotope (232Th), as a rarely occurring isotope, decays to one of the lead isotopes (208Pb) at a constant rate. By measuring intensities of parent and daughter isotopes, the formation age of the stone can be estimated without using ‘time capsule’ inclusions, such as zircon. This can sometimes be helpful, as the zircon inclusions are rarely found to be reaching to the surface of gemstones, hence challenging for age dating by LA-ICP-MS. In an example of a blue sapphire (Figure 2), conventional gemmological testing suggests Madagascar as its origin rather than Myanmar. During routine elemental analysis, rarely occurring 232Th isotope was detected in this sapphire. Thanks to the full mass spectrum acquisition by GemTOF, all of the Pb isotopes (204Pb, 206Pb, 207Pb, 208Pb) were collected simultaneously without re-ablation, and indicated no common Pb contamination. The estimated age (~500Ma) is in agreement with that of Madagascar samples expected in other study (Elmaleh et al. 2015), which adds more evidence to the origin determination.
Multi-Dimension Data Visualization: PCA and t-SNE
Conventionally, trace element results of gemstones are shown in bivariate plots, tri-plots, and three dimensional scatter plots to compare their elemental similarities with reference samples from database. As an example for emeralds, Figures 3a and 3b display a bivariate plot (Li- Cs) and a three dimensional scatter plot (Li-Fe-Cs) using SSEF emerald database. LA-ICP-TOF-MS intrinsically produces multi-element results (high dimensional dataset), therefore one would need to compare multiple bivariate-plots for a comprehensive data analysis, because direct visualization of the high dimensional dataset is challenging. Alternatively, statistical dimension reduction can be applied on the original dataset. Our high dimensional space of twenty element concentrations of more than 700 emerald analyses is projected onto a two dimensional space.
Using this example, linear principle component analysis (PCA) and non-linear machine learning algorithms (t-SNE, Van Der Maaten, 2008) were applied on our datasets (Figures 3c and 3d). Both analyses are unsupervised, meaning colours (indicating various origins) of the scatter dots are labeled only after the reduction process. In this way, groups of data points are solely dependent on the elemental similarities among the analyzed gemstones and without prior information about their origins. Based on our research, we can see that, compared to PCA, we achieve a better separation of different origins when using t-SNE algorithm (Figures 3c and 3d). In this example, the emeralds from different geographical origins can be separated from each other. Emeralds from a new find in Afghanistan (black arrow in Figure 3d), which are gemmologically similar to Colombian material (Krzemnicki, 2017), can also be distinguished from more classic emeralds from Panjshir valley in Afghanistan and Colombia. It seems that non-linear dimension reduction algorithm t-SNE is more suitable for multi-element data visualization comparing to other types of linear algorithms.
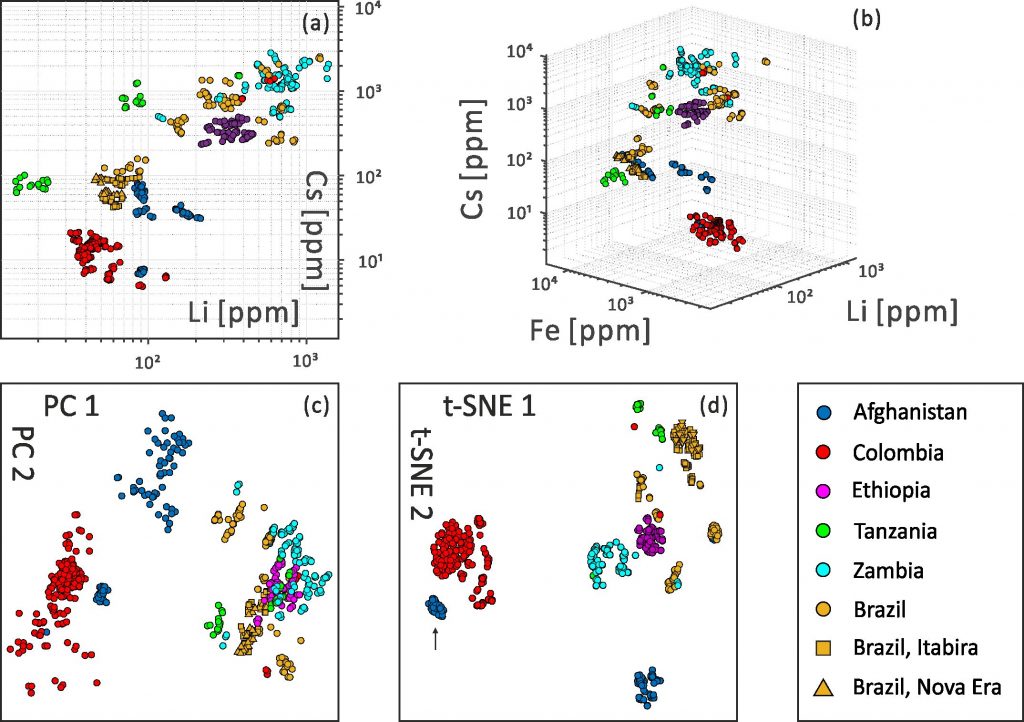
Conclusions
Although the present study reveals the potential of elemental analysis combined with statistical analysis to separate gemstones from different origins and geological settings, it is important to mention that this method is not always conclusive. Especially for corundum, multi-element analysis is rather providing complementary information, to assist microscopic observations and further analyzed properties before concluding on an origin opinion. However, as shown in this study, such statistical methods can be a valuable tool, when studying elemental similarities of gemstones from various origins. Ongoing studies focus on combining elemental data with data from other analytical methods, such as (UV-Vis, FTIR, Raman) spectroscopy and microscopy, with the aim to advance our understanding of the geological conditions during formation of gemstones and finally deepen our knowledge about origin determination of gemstones as a service to the trade.